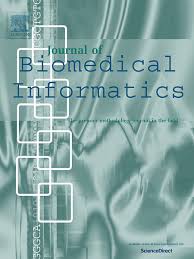
Assessing racial bias in healthcare predictive models: Practical lessons from an empirical evaluation of 30-day hospital readmission models
Jonathan Weiner, Hadi Kharrazi, H Echo Wang, Suchi Saria, Harold Lehmann
Despite increased availability of methodologies to identify algorithmic bias, the operationalization of bias evaluation for healthcare predictive models is still limited. Therefore, this study proposes a process for bias evaluation through an empirical assessment of common hospital readmission models. The process includes selecting bias measures, interpretation, determining disparity impact and potential mitigations. This study proposes an Applied Framework to Assess Fairness of Predictive Models (AFAFPM) and demonstrates the process using 30-day hospital readmission model as the example. It suggests the feasibility of applying algorithmic bias assessment to determine optimized risk thresholds so that predictive models can be used more equitably and accurately. It is evident that a combination of qualitative and quantitative methods and a multidisciplinary team are necessary to identify, understand and respond to algorithm bias in real-world healthcare settings. Users should also apply multiple bias measures to ensure a more comprehensive, tailored, and balanced view. The results of bias measures, however, must be interpreted with caution and consider the larger operational, clinical, and policy context.
Wang HE, Weiner JP, Saria S, Lehmann H, Kharrazi H. Assessing racial bias in healthcare predictive models: Practical lessons from an empirical evaluation of 30-day hospital readmission models. J Biomed Inform. 2024 Aug;156:104683. doi: 10.1016/j.jbi.2024.104683. Epub 2024 Jun 24. PMID: 38925281.