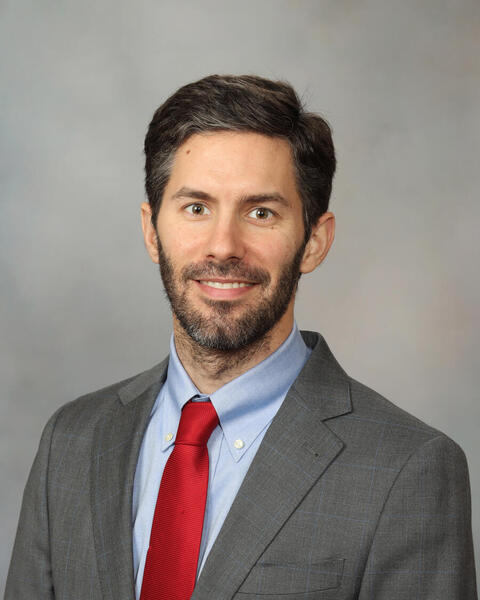
Monthly AI Seminar Synopsis: Implementing AI in ENT Clinical Practice
Featuring Tinglong Dai, Risa Wolf, Christina Yuan
Meeting Summary for HBHI Workgroup on AI and Healthcare (12/13/2024)
Quick Recap
The December seminar of the HBHI Workgroup on AI and Healthcare, moderated by the co-chairs, Dr. Tinglong Dai, Bernard T. Ferrari Professor of Business at Johns Hopkins Carey Business School, and Dr. Risa Wolf, Associate Professor of Pediatric Endocrinology at Johns Hopkins School of Medicine, featured Dr. Santiago Romero-Brufau, Director of AI and Systems Engineering in the Department of Otolaryngology — Head and Neck Surgery at Mayo Clinic. The session focused on the integration of artificial intelligence (AI) into clinical practice, particularly in otolaryngology, highlighting innovations in triage workflows, Large Language Models (LLMs) for document automation, and strategies for balancing false-positive and false-negative outcomes. Discussant Dr. Christina Yuan added implementation science perspectives, addressing equity, user engagement, and sustainability in healthcare AI.
Summary
AI in Otolaryngology Practice
Dr. Santiago Romero-Brufau presented Mayo Clinic’s work in integrating AI into clinical workflows, particularly for managing chronic dizziness, a condition that often requires expertise from ENT, neurology, audiology, behavioral medicine, and physical therapy. Chronic dizziness affects an estimated 70 million Americans, and at Mayo Clinic, managing these patients involves complex multi-specialty workflows.
The traditional triage process at Mayo Clinic was inefficient, with clinicians spending 20-40% of their appointment time reviewing patient referrals. This manual process created bottlenecks, delayed patient care, and constrained the clinic’s ability to accommodate new patients. To address these issues, Mayo implemented an AI-driven triage system, which automated parts of the process while preserving clinician oversight.
The system replaced paper-based workflows with an adaptive electronic questionnaire, ensuring patients only answered questions relevant to their symptoms. For example, if a patient reported dizziness, the system would ask targeted follow-ups related to balance or vertigo. The AI used a decision-tree model to predict appropriate appointments, achieving 87% accuracy in historical data. Clinicians could review and adjust AI recommendations before finalizing patient schedules, ensuring adaptability and trust.
The new workflow resulted in a 75% reduction in triage time, allowing Mayo Clinic to increase patient throughput without adding staff. This hybrid approach, combining automation with clinician oversight, exemplifies how AI can optimize healthcare operations while maintaining quality and trust.
Framework for Scaling AI in Healthcare
Dr. Romero-Brufau introduced a six-step framework for scaling AI tools in clinical settings, inspired by NASA’s technology readiness model. This framework ensures AI models transition effectively from research to clinical practice:
- System Redesign: Adjust workflows to integrate AI tools without disrupting existing processes.
- Data Quality: Collect and preprocess high-quality, real-time, structured data for model training and deployment.
- Model Selection: Use interpretable models that clinicians can adapt to specific needs.
- Human-Computer Synergy: Define the complementary roles of AI and clinicians, leveraging their respective strengths.
- Change Management: Engage stakeholders early to build trust, refine workflows, and ensure successful adoption.
- Result Measurement: Validate success through metrics such as time savings, resource efficiency, and patient outcomes.
This framework guided the implementation of the chronic dizziness triage system, ensuring alignment with operational needs and clinical workflows.
Balancing Operations, Availability, and Model Design
One of the challenges in triage is ensuring that AI recommendations align with resource availability and patient needs. Dr. Romero-Brufau explained how Mayo optimized operational workflows by dynamically adjusting the thresholds for the AI model’s recommendations based on the availability of specialty appointments. For example:
- Neurology Appointments: When neurology slots were limited, the model erred on the side of reducing false positives, prioritizing patients whose symptoms strongly indicated the need for neurological evaluation.
- Flexible Scheduling: If a patient’s need for a specialty was uncertain, they were still scheduled for other appointments, with the option to add the specialty visit later.
This adaptability allowed the system to balance triage accuracy with operational realities, ensuring efficient use of limited resources without sacrificing patient care quality.
LLMs for Clinical Documentation
Mayo Clinic is piloting Large Language Models (LLMs) to automate information extraction from clinical documents, reducing the cognitive burden on clinicians. The workflow for these projects includes identifying patient cohorts, selecting relevant clinical documents, using LLMs to extract key information, and validating outputs against manually reviewed data.
Key applications include:
- Automating chart reviews for research and quality improvement.
- Predicting cochlear implant eligibility.
- Identifying surgical candidates for chronic sinusitis.
Dr. Romero-Brufau noted that while commercial LLMs have performed well, they require rigorous validation to ensure reliability, fairness, and equity.
Implementation Science Perspectives
Discussant Dr. Christina Yuan emphasized the critical role of implementation science in ensuring sustainable adoption of AI tools. Key points included:
- User Engagement: Building trust through transparency and incorporating clinician feedback into system design.
- Equity: Addressing socio-economic disparities and health literacy to ensure AI tools are accessible to all patient populations.
- Sustainability: Moving beyond “train-and-pray” approaches to implementation by studying actions that promote long-term use.
Dr. Yuan stressed that implementation is a deliberate, iterative process requiring close collaboration between clinicians, engineers, and administrators.
Q&A Highlights
- Impact of Workflow Redesign on Data Quality:
A question was raised about how data capture changed during the redesign. Dr. Romero-Brufau explained that transitioning to structured, multiple-choice questionnaires improved data consistency and allowed the AI model to process information more effectively. Open-ended questions, often a barrier to automation, were minimized to streamline operations.
- Balancing False Positives and False Negatives:
A participant asked how the model balanced the cost of misclassification. Dr. Romero-Brufau noted that the model was tuned to minimize false positives when resources like specialty appointments were scarce. However, the workflow design allowed for flexibility—false negatives did not result in missed care but rather deferred specialty visits, ensuring patients received necessary treatment without overwhelming the system.
- Equity in AI Tools:
Concerns were raised about the potential impact of health literacy on AI adoption. Dr. Romero-Brufau acknowledged that while the model performed equitably across race, gender, and ethnicity, health literacy was not specifically studied and remains a critical area for future exploration.
- FDA Approval for AI Systems:
A question about regulatory considerations revealed that the triage system did not require FDA approval, as it was classified as an operational aid rather than a diagnostic device. Internal use and clinician oversight further reduced regulatory complexity.
- Incorporating Decision Analysis into AI:
Participants discussed how decision analysis methods could be integrated into AI models to better balance operational constraints and clinical outcomes. Dr. Romero-Brufau expressed interest in exploring collaborations in this area.
- Use of Asymmetric Cost Functions:
The discussion touched on the potential for incorporating asymmetric cost functions into future AI models to better account for the differing consequences of false positives and false negatives. This approach could improve model alignment with clinical priorities.
Thank you for your support in 2024 — and your continued support in 2025! Happy Holidays! We'll be announcing our January seminar soon! Stay tuned!