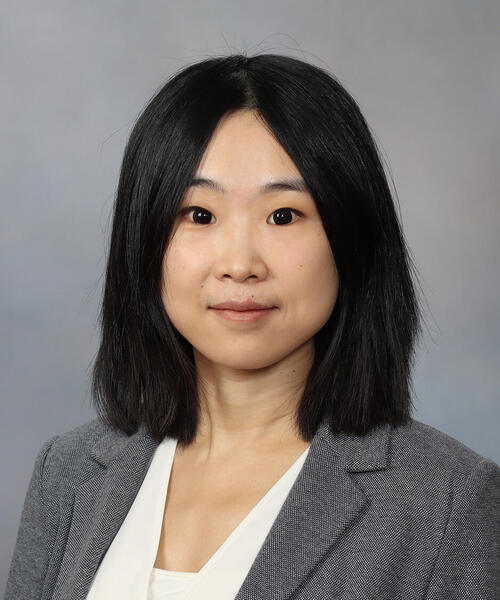
Monthly AI Seminar Synopsis: Mayo Clinic's Pioneering (Gen-)AI Efforts - Part 1
Featuring Tinglong Dai, Risa Wolf
Below is the AI-generated summary of the February 9 seminar, with minor edits made by the AI Workgroup Leadership:
Xiaoxi Yao discussed the evolution of treatment options for high-risk diseases, emphasizing the role of AI algorithms in decision-making. She highlighted the need to consider the difference in risk between different treatment options and the potential of causal machine learning algorithms to assist in treatment selection. Xiaoxi Yao also discussed the evaluation of AI-generated clinical summaries, emphasizing the importance of evaluating the technical performance, usability, and acceptability of AI tools before considering their health impact.
AI in High-Risk Disease Treatment Decisions
Xiaoxi Yao discussed the evolution of treatment options for high-risk diseases, emphasizing the role of AI algorithms in decision-making. She shared her personal experience with high-risk breast cancer and the potential treatment options. Xiaoxi Yao highlighted the need to consider the difference in risk between different treatment options, rather than just the prediction of risk. She also discussed the evaluation of AI algorithms, focusing on metrics such as sensitivity, specificity, positive and negative predictive values, and the need for a threshold for ordering further testing. Xiaoxi Yao also introduced the potential of causal machine learning algorithms to assist in treatment selection and emphasized the limitations of group analysis in clinical decision making.
Large Language Models in Healthcare Summaries
Xiaoxi Yao discussed the topic of large language models, highlighting their increasing popularity in the healthcare sector. She referenced a seminar by Dr. Steve Hart on the subject, available on YouTube, and mentioned an upcoming talk by Jama's Associate Editor. Xiaoxi Yao underscored the importance of evaluating the performance of these models, particularly in their use in healthcare for tasks such as clinical summaries. She suggested that the accuracy and conciseness of the summaries generated by these models should be the focus of their evaluation.
AI Clinical Summary Evaluation Discussed
Xiaoxi Yao discussed the evaluation of AI generated clinical summaries. She highlighted the potential for misinterpretation due to the way information is organized and presented, but emphasized that these should not pose a significant problem. Xiaoxi Yao also outlined the next stage of AI evaluation, focusing on usability and acceptability, using a technology assistance model to assess perceived ease of use, usefulness, and intention to use. She also mentioned using the XAI scale to measure trust in AI and discussed the importance of human review in the workflow.
Evaluating AI Health Impact: Technical, Usability, Acceptability
Xiaoxi Yao discussed the importance of evaluating the technical performance, usability, and acceptability of AI tools before considering their health impact. Xiaoxi Yao emphasized that neglecting these factors could result in a tool that is not used and thus have no impact. Xiaoxi Yao also explained the concept of health services research and how it relates to evaluating the impact of health technologies like AI. Xiaoxi Yao further discussed the methods of evaluating impact, including randomized controlled trials and non-randomized studies. Xiaoxi Yao used the example of the EAGLE trial, which assessed whether AI improves patient care in everyday practice, and the Beagle trial, which evaluated a different AI tool in a decentralized, non-randomized setting.
Digital Solutions and AI in Healthcare Research
Xiaoxi Yao discussed the use of digital solutions and AI in healthcare research. He mentioned the successful implementation of remote enrollment and data collection in a study using their platform, demonstrating the efficiency of these digital tools in research. Xiaoxi Yao also highlighted the potential of the newly developed app which can enroll patients, collect data from wearable devices, and link to electronic health records. Nestoras Mathioudakis responded by discussing the challenges and advantages of using large language models in healthcare documentation. He raised concerns about the potential loss of discrete data for population health tracking and the lack of education on documentation standards for clinicians.
AI and Clinical Decision Support: Challenges and Potential
Nestoras Mathioudakis discussed the potential of using AI technology to standardize clinical documentation and enhance clinical decision support tools. He suggested the possibility of real-time interaction between clinicians and AI models to improve the accuracy of these models. Xiaoxi Yao responded, sharing her experience with the challenges of getting clinicians to react to AI predictions in real time. She pointed out that while some clinicians ignore the AI predictions, others do not act on them due to patient-related factors such as social determinants of health. The discussion concluded without a clear resolution on how to enhance the interaction between AI and clinicians.
Language and AI Model Performance in Clinical Decision Support
Xiaoxi Yao and Nestoras Mathioudakis discussed the importance of language in clinical decision support tools and the need for further research in this area. Nestoras suggested that changing the tone of the language could influence the provider's scoring of the recommendation's effectiveness or safety. Gordon Gao emphasized that while model performance is important, the impact of AI is determined by multiple factors, including user acceptance. Gordon Gao raised the question of whether there is too much emphasis on model performance, suggesting a need to balance the development and testing of AI models with their real-world implementation.
Algorithm Performance, Clinician Capacity, and Ethical Implications
Xiaoxi Yao stressed the importance of assessing the performance of algorithms before implementation, emphasizing that they must outperform existing models. Nestoras Mathioudakis raised concerns about the gap in understanding of how many decision support systems a clinician can handle. Risa Wolf highlighted the need to consider the capacity of diagnostics and treatment when using AI-based screening and the ethical implications of such use. The group also discussed the challenges of implementing and maintaining these systems, with Xiaoxi Yao explaining the process of a randomized controlled trial they conducted. Peter Greene concluded by asking about the management of rapidly changing models, to which Xiaoxi Yao responded that stakeholder engagement and continuous improvement are key.