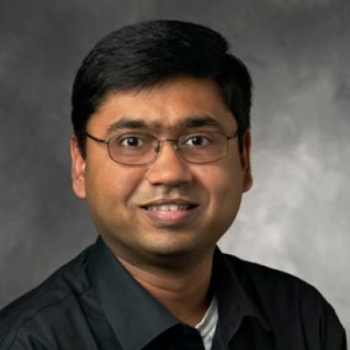
Monthly AI Seminar Synopsis: Shaping the Responsible Use of AI
Featuring Tinglong Dai, Risa Wolf
Meeting summary for HBHI Workgroup on AI and Healthcare (09/13/2024)
Quick recap:
The meeting focused on the responsible use of AI in healthcare, emphasizing the need for sustainable integration into clinical practice. Featured speaker Dr. Nigam Shah from Stanford discussed the importance of aligning AI models with healthcare realities, particularly around capacity constraints and financial sustainability. The session also covered ethical issues, such as addressing unconscious bias in AI decision-making, and explored strategies to improve data usage, workflow design, and incentive structures in AI implementation.
Key discussion points:
AI in Healthcare: Integration and Sustainability
Dr. Nigam Shah emphasized the responsible and ethical integration of AI in healthcare, stressing the importance of bridging the academic-business divide to make AI scalable and sustainable. He introduced patient timelines as the foundation for AI decision-making, urging that the conversation around AI must start with data and focus on how AI models can assist clinicians in diagnoses and treatment recommendations.
Shah highlighted the importance of stakeholder exchange rates, where different stakeholders make trade-offs, such as surgeons weighing how many unnecessary biopsies are acceptable to catch one cancer case. These exchange rates guide decisions on how AI models should set thresholds for actionable outcomes.
Challenges in AI Model Implementation
Shah identified major hurdles in AI adoption, such as capacity constraints and the unsustainable costs of multi-site model validation efforts. He shared an example of a $28 million, 10-year effort to validate a model across several sites, emphasizing that AI needs to be developed in a way that does not exhaust healthcare resources.
He also discussed how feasibility testing and the evaluation of achievable benefits must be integral steps in AI development to avoid the trap of "pilotitis," where pilot projects are endlessly run without scaling them for real-world use.
The Role of Data in AI: Patient Timelines and Decision-Making
Shah emphasized that most AI tasks in healthcare are actually classifications, though they are often mistakenly referred to as predictions. Using the example of heart failure, Shah explained how AI can help uncover disease subtypes and how these insights can improve both the science and practice of medicine. However, the true challenge lies in moving these advances into real-world healthcare delivery.
Evaluating AI Models and Addressing Bias
Shah pointed out that most current evaluations of language models in healthcare do not utilize EHR data, limiting their practical applicability. He advocated for defining AI benefits upfront and ensuring they are verified throughout implementation to prevent waste.
Shah also raised concerns about unconscious bias in AI models, referencing a case where GPT-3.5 and GPT-4 were used to answer bedside medical questions. While clinicians agreed more often with AI-generated responses from GPT-4, disagreements still occurred 50% of the time, illustrating the complexities of applying language models in medical practice.
Language Models for Patient Responses and Time-to-Event Training
Shah discussed how language models used for patient responses did not significantly improve productivity in studies from Stanford, UC San Diego, and Mayo Clinic. However, they did reduce the cognitive burden on physicians, offering potential benefits in wellness rather than efficiency.
He also introduced time-to-event training, which allows AI models to be trained with minimal data, making it particularly useful for rare disease classification. These models demonstrated better performance and adaptability across sites, with reduced data requirements.
AI in Cost-Effectiveness and Incentives
Dr. Antonio Trujillo discussed the economic implications of AI, particularly the need for allocative efficiency—ensuring AI solutions are deployed where they can have the greatest impact. He raised concerns about incentive alignment, stressing the importance of ensuring that AI technologies are both cost-effective and equitably distributed across healthcare systems.
Q&A
Dr. David Newman-Toker, a neurologist at Johns Hopkins, asked whether the challenges of AI deployment were primarily AI problems or implementation science problems. He highlighted the difficulties in changing workflows and perceptions in healthcare. Shah responded by proposing that implementation science must expand into delivery science, focusing on both upstream intervention design and downstream execution. Dr. Kathy McDonald followed up by asking how data strategy impacts the deployment of AI models, to which Shah explained that data storage and compute costs are often underestimated in project planning. He argued that these logistical concerns should be considered earlier in the model development process. Dr. Gordon Gao asked if certain healthcare organizations are better equipped to adopt AI technologies. Shah responded that while some institutions like Hopkins, Duke, Stanford, and Vanderbilt have made strides, there has been no rigorous research to rank these organizations by their AI readiness. Much of the success depends on local incentive structures and organizational priorities.
Dr. Peter Greene raised a question about the efficacy of assurance checklists, asking whether they could accelerate AI deployment. Shah expressed skepticism about the usefulness of more PDFs but noted that checklists are a necessary first step to identify and simplify the key challenges. He argued that transforming these checklists into software tools would be the real driver for faster, more effective implementation.
Closing Remarks
Drs. Tinglong Dai and Risa Wolf wrapped up the seminar, thanking Dr. Shah for his insightful presentation and discussion. They announced that the next HBHI seminar will be held on October 11, 2024, featuring Dr. Charlotte J. Haug. (Note: This is a correction from the announcement made in the seminar.)