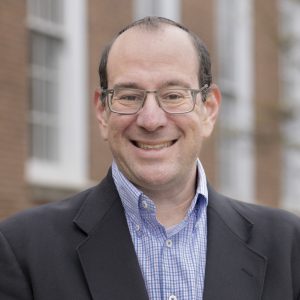
Monthly AI Seminar Synopsis: Large Language Models in Medicine
Featuring Tinglong Dai, Risa Wolf
Seminar Summary for HBHI Workgroup on AI and Healthcare (11/08/2024), Featuring Dr. Mark H. Dredze
Speaker Bio: Dr. Mark H. Dredze, PhD, is the Interim Deputy Director of the Data Science and AI Institute and the John C. Malone Professor in the Department of Computer Science at Johns Hopkins University. He also serves as the Associate Head of Research and Strategic Initiatives within the department. Dr. Dredze’s research focuses on artificial intelligence and natural language processing (NLP) with applications in public health, clinical informatics, and social media analysis. His work includes developing NLP methods for tasks such as information extraction and sentiment analysis, and he has contributed to public health initiatives involving tobacco control, vaccination, infectious disease surveillance, mental health, and gun violence prevention. Dr. Dredze is affiliated with the Malone Center for Engineering in Healthcare and holds a joint appointment in the School of Medicine's Biomedical Informatics & Data Science Section.
Abstract: Dr. Mark H. Dredze’s presentation, titled “Large Language Models in Medicine: Opportunities and Challenges,” explored the significant advancements in generative AI, particularly focusing on large language models (LLMs). He provided a comprehensive overview of how LLMs have evolved from early research to the sophisticated systems in use today, emphasizing the transformative impact of their large-scale implementation. Dr. Dredze highlighted the capabilities of these models in medical applications, discussed their potential and limitations, and outlined the challenges of evaluating AI tools in a clinical context. He also addressed concerns related to cultural biases, patient safety, and regulatory hurdles, stressing the importance of interdisciplinary collaboration in advancing these technologies responsibly.
Summary:
(HBHI) hosted a seminar as part of its AI and healthcare series. The event featured Dr. Mark H. Dredze from Johns Hopkins University, moderated by Dr. Tinglong Dai and Dr. Risa Wolf. The seminar drew a diverse audience of healthcare professionals, researchers, trainees, and AI experts to discuss the progress and challenges involved in applying AI, specifically large language models, to healthcare.
Dr. Dredze began by tracing the history of language models to research in the 1970s, emphasizing the foundational contributions made at Johns Hopkins by figures such as Fred Jelinek. He noted that while language models have existed for decades, the leap to current capabilities has come through the massive scale at which these models are now trained. Today’s LLMs, such as those that power ChatGPT, consist of hundreds of billions of parameters and are trained on datasets containing trillions of tokens. This scaling has enabled these models to generate complex, human-like language, allowing for impressive applications in various fields, including healthcare. While ChatGPT gained public attention in 2022, Dr. Dredze pointed out that similar technologies had been under academic exploration since 2020.
The potential for LLMs in medical applications is significant. Dr. Dredze described how these models can be used to draft medical records, support patient communication, and assist with diagnostic decision-making. He noted that, despite their capabilities, LLMs should complement rather than replace clinicians. Their value lies in their ability to help medical professionals process large volumes of data and generate insights efficiently.
A central theme of the presentation was the challenge of evaluating AI in clinical settings. Traditional methods used for machine translation are inadequate for the nuanced and complex nature of medical language. Dr. Dredze advocated for expanding evaluation criteria to include factors such as empathy, authenticity, and thoroughness, ensuring that AI-generated responses meet the standards of patient care. He highlighted the difficulty of applying the slow, rigorous clinical trial process to rapidly evolving AI technologies, noting that regulatory bodies like the FDA face challenges in adapting to the fast pace of AI development.
Dr. Jodi Segal, serving as the discussant, brought a clinical perspective to the conversation. She emphasized the need for AI tools to be developed with input from healthcare providers to ensure they meet real-world medical needs and maintain patient safety. Dr. Segal also discussed the importance of fostering collaboration between clinician-researchers and AI experts to leverage large datasets more effectively in healthcare.
The seminar featured a discussion on the potential of AI tools like ChatGPT in enhancing patient care. Dr. Dredze shared findings from a study comparing ChatGPT’s responses to patient questions with those from licensed physicians.
The results indicated that the AI offered more empathetic and detailed responses, showcasing its potential to improve patient interactions. However, he cautioned that AI models, while powerful, are not infallible and must be carefully evaluated before being integrated into medical practice.
During the Q&A session, Dr. Dredze addressed the common issue of AI systems appearing overly confident. He explained that this stems from training data that often includes assertive human-written content, leading models to project confidence even when expressing uncertainty would be more appropriate. Raj Vadigepalli raised concerns about the ability of current models to handle cultural nuances and non-English outputs, which Dr. Dredze acknowledged as a limitation due to the English-centric nature of most AI research. He called for more diverse training data to improve the inclusivity and applicability of AI in various healthcare contexts.
Dr. Dredze also touched on the potential for LLMs to simplify complex medical documents and aid patients in understanding their care. He discussed the role of AI avatars in medical education, which could offer interactive learning experiences for students and practitioners. However, he noted that while these avatars can simulate human interaction, they often lack the deeper understanding needed for complex decision-making.
Regulatory and ethical considerations were a significant part of the discussion. Dr. Dredze highlighted the challenges of aligning fast-evolving AI technologies with existing regulations and emphasized the importance of transparency, accountability, and fairness. Dr. Segal and other participants, such as Daniel Byrne, suggested that randomized controlled trials comparing AI-augmented care with standard practices could provide a more effective way to evaluate AI’s impact on patient outcomes.
The seminar concluded with Dr. Dredze expressing optimism about ongoing efforts at Johns Hopkins to develop HIPAA-compliant, user-friendly AI tools that could empower both researchers and clinicians.